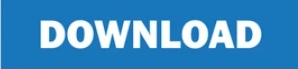


Īnciaes PR, Jones P (2018) Estimating preferences for different types of pedestrian crossing facilities. Īl-Janabi S, Mohammad M, Al-Sultan A (2020b) A new method for prediction of air pollution based on intelligent computation. Īl-Janabi S, Alkaim AF, Adel Z (2020a) An Innovative synthesis of deep learning techniques (DCapsNet & DCOM) for generation electrical renewable energy from wind energy. Īl-Janabi S, Salman MS, Fanfakh A (2018) Recommendation system to improve time management for people in education environments. Īl-Janabi S, Al-Shourbaji I (2016) A study of cyber security awareness in educational environment in the middle east. Īl-Janabi S, Alkaim AF (2020) A nifty collaborative analysis to predicting a novel tool (DRFLLS) for missing values estimation. Īlam MD, Rahman MS, Rahman MS (2019) A random forest based predictor for medical data classification using feature ranking. Civ Environ Res 3(1):66–79Īlajali W, Zhou W, Wen S, Wang Y (2018) Intersection traffic prediction using decision tree models. Multivariate adaptive regression splines E-CHAID:Ĭhi-squared automatic interaction detector SVM:īack propagation neural network DCapsNet:ĭetermined structure network-particle swarm SAQPM:Ībojaradeh M (2013) Evaluation of pedestrian bridges and pedestrian safety in Jordan. Random forest regression and classification BTCR:īoosted tree classifiers and regression MARS: Symmetric mean absolute percentage error MAD:Ĭlassification and regression trees RFRC: Receiver operating characteristic curve SMAPE: Normalized root-mean-squared error AUROC:Īrea under receiver operating characteristics curve AUPR: The identification of important variables not only provides better insight of factors that affects the choice of pedestrians using the elevated facilities but also provides a valuable source of information to researchers, planners and policymakers to construct a better-planned pedestrian friendly infrastructure.ĭevelopment of the long short-term memory PTT: Therefore, provision of CCTV cameras and security personnel, removal of obstruction, provision of proper lighting and all-weather shade, and regular maintenance of the facilities will significantly improve the pedestrians’ choice to use the FOBs. The safety and security, walk environment, frequency of daily use, comfort, location type, length of travel, stairway dimensions and reduced walkable width affected the choice of using the FOBs. Further, the respondents’ feedbacks regarding existing problems were used to validate the findings. Sensitivity analysis supported the fact that gender and age had significant impact on the choice of pedestrians under different contexts. GBM helped in identifying the essential parameters that drive the usability of FOBs under the four different contexts. The study results revealed that GBM algorithm showed highest classification accuracy on test dataset over the other two techniques at various scenarios. Finally, the developed models were evaluated for test dataset for generalization. The next stage involved splitting the data into training and testing set, followed by model training and hyper-parameter optimization using tenfold cross-validation. The modelling approach involved data collection from 14 FOB locations, preprocessing involving data input in spreadsheets, removing missing values and normalization for model training.

Three soft computing algorithms such as generalized linear model (GLM), random forest (RF), and gradient boosting machine (GBM) were trained to predict the future usability of pedestrians.
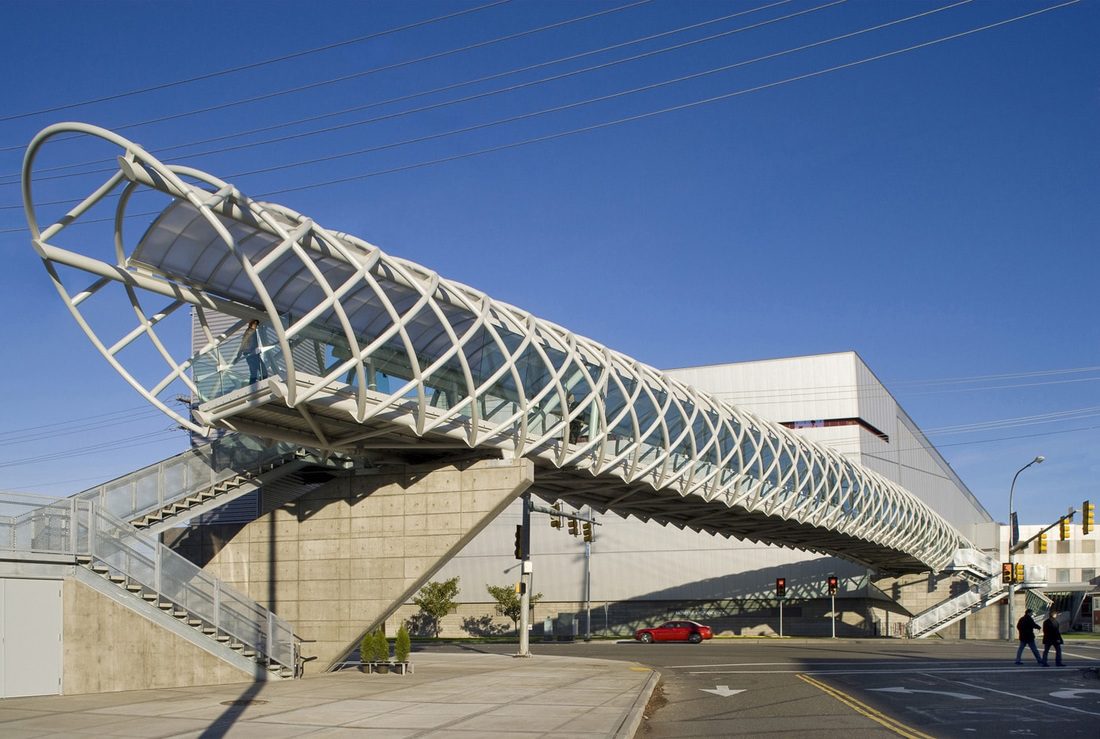
The goal was to identify the essential features that drive the usability of FOBs under four different contexts, i.e., mobility friction, safety and security, and vertical connectivity and horizontal connectivity. The study utilized both questionnaire survey (perception in terms of satisfaction/dissatisfaction) and field data collected across fourteen locations in six different Indian cities. The aim of the work presented here was to identify the important predictors that determine usability of FOBs. There is paucity of studies that uses modern soft computing techniques to understand the factors driving the use of FOBs. The identification, understanding, and treatment of predictors that drive the use of pedestrian overpass or Foot Over Bridge (FOB) are very much essential for city planners and policymakers.
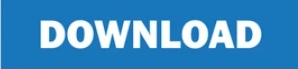